Dr. Zou and his team developed an AI system that can search Twitter for images of skin lesions and provide potential diagnoses. This allows dermatologists to compare their cases to similar ones shared on social media. The system was trained on over 100,000 images of skin conditions tweeted by dermatologists. It can match new lesion images to visually similar ones in its database and provide the related diagnoses.
Dr. Zou believes that Obtaining an ample amount of data poses a critical challenge for advancing medical AI applications. His research demonstrates an innovative approach that leverages the vast reservoir of medical insights openly shared on social media platforms to construct robust models.
To ensure the integrity of the data from Twitter, they employed a series of filters, ultimately enhancing its quality. Their method, known as PLIP (Prescribed Label Inference Paradigm), exhibits exceptional performance across various tasks, including zero-shot classifications, fine-tuning procedures, as well as image and text-based retrievals.
This approach has great potential to facilitate knowledge sharing in the medical field. Social media contains a wealth of data that can be tapped into to improve AI systems. The data is more diverse, representing different demographics and geographic locations. This can make the AI more equitable and help it provide better diagnoses for underserved communities.
The researchers recognize that recent changes at Twitter regarding data access could be a potential challenge. However, Dr. Zou believes the core message of their research remains valid beyond any one social media platform. There are creative ways to obtain data from diverse sources to improve medical AI. This could include forming partnerships with healthcare organizations to get access to their images and data.
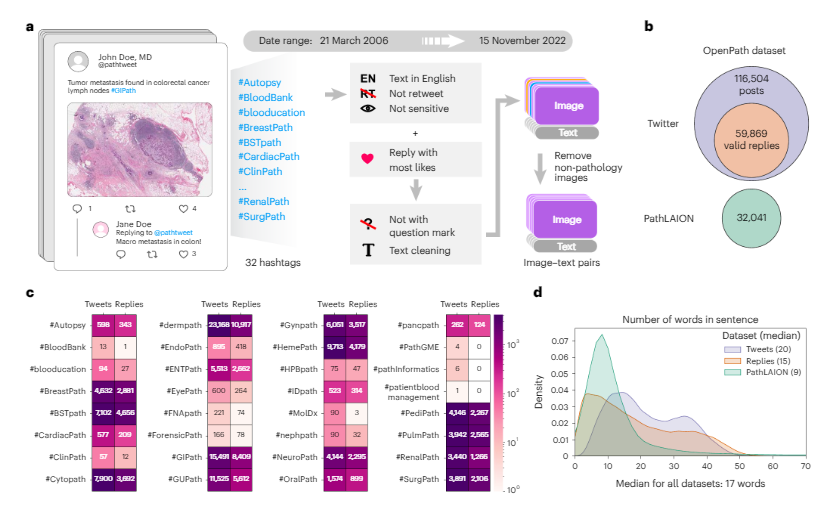
Overall, this work demonstrates the power of combining AI and social media data to advance medicine. The applications could extend beyond dermatology into other fields like radiology, pathology, and ophthalmology. As long as access to data can be obtained ethically, the approaches explored here have great potential to make AI systems more accurate, equitable and globally effective at assisting doctors. This could lead to improved healthcare access and outcomes around the world.
The our data (OpenPath): https://drive.google.com/drive/folders/1b5UT8BzUphkHZavRG-fmiyY9JWYIWZER
The paper can be freely accessed (w/o paywall) here: https://www.nature.com/articles/s41591-023-02504-3.epdf
All our data (OpenPath):